Bioinformatics (Milan)
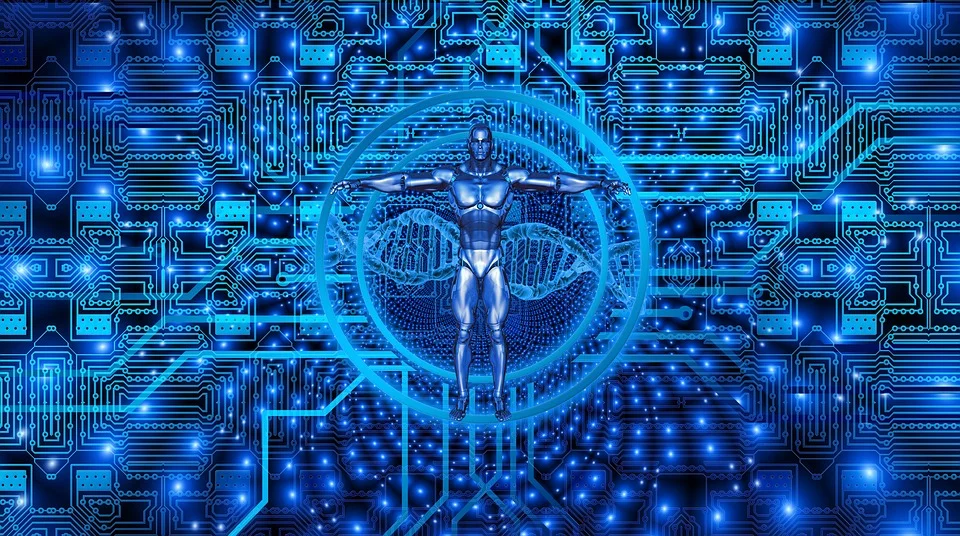
The Bioinformatics Lab consists of approximately 15 researchers and technologists having a diverse background encompassing bioinformatics, biotechnology, engineering, physics and computer science.
The Lab develops, implements and applies advanced analysis techniques in order to tackle open problems from the Life Sciences realm, with particular attention to key sectors such as genomics, epigenomics, bulk and single-cell transcriptomics, multi-omics data integration, molecular biodiversity, systems biology, molecular modelling and artificial intelligence.
Additionally, biological data banks and bioinformatics tools are designed and developed within the Lab for Big Data analyses in the sectors of biomedicine, nutrition and environmental problems. A Translational Bioinformatics unit also part of this Lab provides preparation of biological samples and performs related *omics analyses so to further support and validate the bioinformatics predictions.
The Lab has built and manages an advanced HPC (High Performance Computing) infrastructure so to support the Lab projects’ computational needs from the inside. The infrastructure provides predictable execution times for the computations without needing to rely on external resources.
Research Topics
The Lab has gained experience in the study of gene-environment interaction in the onset of autism. It is involved, with other European and US partners, in the EU project “Genome, environment, microbiome and metabolome in autism” (GEMMA). GEMMA aims to perform multi-omics analyses on biological samples of newborns at risk of developing autism and collected at different time points. The integration of omics and clinical data will identify biomarkers associated with autism useful for personalized therapies.
In the framework of the collaboration program with the San Raffaele Hospital and the Telethon Foundation, established for studying the safety of Gene Therapy, the Lab has developed great experience in data analysis concerning regenerative medicine. In particular, we have proficiency in the application of bioinformatic approaches to the study of biological mechanisms related to monogenic diseases treatable through gene delivery with lentiviral vectors and in data analysis both for developing preclinical models and for clinical post-treatment monitoring using NGS techniques.
We actively collaborate to the improvement of Gene Therapy through computational approaches, both for developing safer and more efficient viral vectors, and for controlling inflammatory and senescence processes in human hematopoietic stem cells to transplant. The Lab has also developed novel approaches for the data analysis in the context of CRISPR gene editing and cancer immunotherapy through Chimeric Antigen Receptor T cells.
ur Lab has extensive experience in the functional study of macromolecules at the atomic level to get a better understanding of the molecular processes, recognition and binding mechanisms of macromolecules responsible for human diseases. Molecular modeling, docking, molecular dynamics and bioinformatics are applied for the study of macromolecular structures (protein, RNA and DNA) and performed on high performance computing (HPC) infrastructures. We have developed skills for the identification or repositioning of selective and high affinity compounds, new leads or known drugs, that tightly bind therapeutic targets by applying virtual high-throughput screening (VHTS) methods. Our analyses have been applied in the contexts of Cystic Fibrosis, Covid19, HIV and Endocrine Disruptors, mutants of the coagulation cascade, proteins involved in the evolution of cancer or in the celiac disease, and rare diseases.
Methods for the analysis of multiple layers of biological information pave the way for a more comprehensive and deeper understanding of biological systems. The complexity of biological systems, the technological limits, the large number of biological variables and the relatively low number of biological samples make integrative analyses a challenging issue. Hence, the development of methods for the integrative analysis of multi-layer datasets is one of the most relevant problems computational scientists are addressing nowadays.
A relevant insight for translating gene-level findings into a mechanistic understanding of disease pathogenesis and more effective strategies for prevention, diagnosis, and treatment is that gene products do not act independently, but in relation with a complex network of other players. We develop methods to jointly analyse the interactome and single or multiple omics datasets in order to capture patterns (e.g., active gene networks, disease modules) that can explain the relation between perturbations and phenotypic disease manifestations. Our tools are aimed at extracting knowledge for descriptive and/or predictive purposes.
STAFF
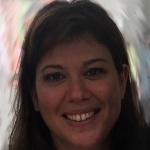
Federica Chiappori
Researcher
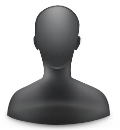
Francesca Cupaioli
Researcher
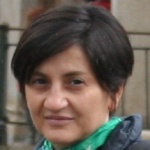
Pasqualina D'Ursi
Researcher
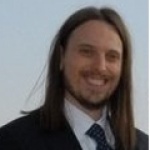
Matteo Gnocchi
Technologist
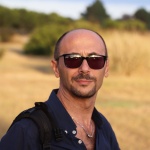
Andrea Manconi
Researcher
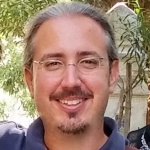
Ivan Merelli
Researcher
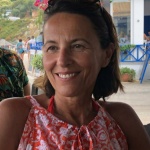
Alessandra Mezzelani
Researcher
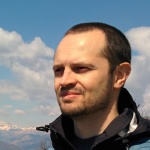
Ettore Mosca
Researcher
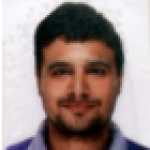
Marco Moscatelli
Technologist
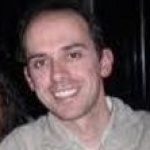
Alessandro Orro
Researcher
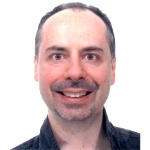
Gabriele Antonio Trombetti
Researcher
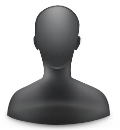
Matteo Uggeri
Scholarhip Fellow
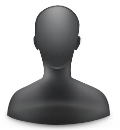
John Hatton
Technician
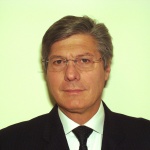
Luciano MIlanesi
Associated Researcher